
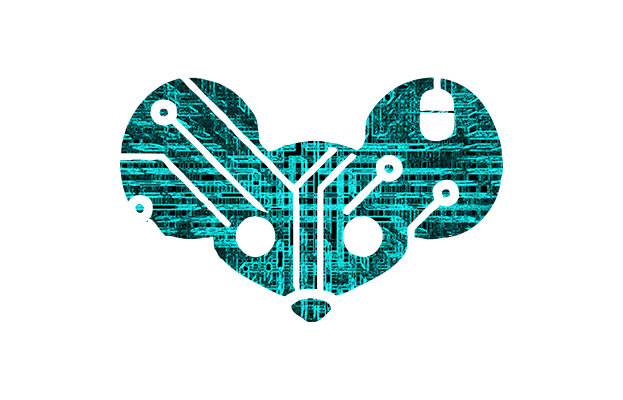
Turns out land is still cheap and sunlight still generally free.
“The future ain’t what it used to be.”
-Yogi Berra
Turns out land is still cheap and sunlight still generally free.
The universe originated as a vibrational wave from Fred Flintstone playing a dinosaur bone keyboard.
tits out for harambe
I have two of those cats. I still can’t catch them when its time to go to bed.
You don’t owe anything to anyones prudishness.
“It’s crucial…”
Think of inflation from monetary policy like lead in the environment. It takes time, but it all eventually ends up in the bones. Real property are those bones. 13 trillion dollars were ‘created’ during covid. Inflation (which i don’t think is necessarily the right word to use, because it implies it can go backwards), takes time to get “into” the price of products, but products that transact faster, inflate faster. Property “inflates” on a relatively long time horizon, but its often very substantial appearing jumps because the transaction cycle is so long (typically 10+ years between transactions).
So maybe you’ve not bought a home, but try not to think about things in the absolute value of their price-tag, but rather in the monthly cost of the money to make the payments on the debt. At 2.5% interest, lots of people can afford a 500k house. At 6% interest far fewer can. If the seller re-financed at less than inflation, its literally more “profitable” to just pay the mortgage than to sell.
I see some others blaming air-bnb’s. There is very little evidence for this. A few papers have found significant correlations, but the effect is so small relative to inflation that we should probably ignore it, and most of these papers are from a pre-inflationary period. Orders of magnitude more impactful is the fact that so many home owners were able to either purchase or re-finance at an extremely low rate in 2020/2021. Blaming Air-bnb’s and ignoring the broader macro-environment in which all property sit is just extraordinarily lazy, reactionary thinking. Air-bnb’s represent a microscopic number of actual rentals, and have been dropping in numbers for years.
new. no filters. it’s like drinking from a garden hose.
my life is spyroing out of contol
I’ve always found earing and necklace sets that they enjoy (they don’t have to be expensive) and are fun to wear are always a hit. Its also a good way to test the water with something relatively cheap (>$50- $100) to identify their style. If possible, I would strongly recommend buying in-person at something like a craft market or farmers market: anything in-person. How something looks online often just does not translate to in-person.
The key here is a set, something they can add in-combination to something they already have.
I’ve done several AI/ ML projects at nation/ state/ landscape scale. I work mostly on issues that can be solved or at least, goals that can be worked towards using computer vision questions, but I also do all kinds of other ml stuff.
So one example is a project I did for this group: https://www.swfwmd.state.fl.us/resources/data-maps
Southwest Florida water management district (aka “Swiftmud”). They had been doing manual updates to a land-cover/ land use map, and wanted something more consistent, automated, and faster. Several thousands of square miles under their management, and they needed annual updates regarding how land was being used/ what cover type or condition it was in. I developed a hybrid approach using random forest, super-pixels, and UNET’s to look for regions of likely change, and then to try and identify the “to” and “from” classes of change. I’m pretty sure my data products and methods are still in use largely as I developed them. I built those out right on the back of UNET’s becoming the backbone of modern image analysis (think early 2016), which is why we still had some RF in there (dating myself).
Another project I did was for State of California. I developed both the computer vision and statistical approaches for estimating outdoor water use for almost all residential properties in the state. These numbers I think are still in-use today (in-fact I know they are), and haven’t been updated since I developed them. That project was at a 1sq foot pixel resolution and was just about wall-to-wall mapping for the entire state, effectively putting down an estimate for every single scrap of turf grass in the state, and if California was going to allocate water budget for you or not. So if you got a nasty-gram from the water company about irrigation, my bad.
These days I work on a small team focused on identifying features relevant for wildfire risk. I’m trying to see if I can put together a short video of what I’m working on right now as i post this.
Example, fresh of the presses for some random house in California:
Your argument is a false dichotomy.
You should look up what a false dichotomy is. A false dichotomy is typically when someone presents two choices as the only possible options, ignoring other possibilities. My argument doesn’t do that. I’m arguing you have no-idea where Stein voters (or Johnson voters for that matter) would go if not for Stein. Also, you may not have noticed it, but you quite literally engage in false dichotomy in your response.
You are still making the assumption that voters only have two choices. No matter how much you’ve convinced yourself that’s the case; its not reality. Voters don’t have to vote. Voters can vote Republican or however they want. No candidate is owed a vote, however much Democrats want that to be a thing.
The entire rhetorical approach you are engaging in is why Kamala has been slipping in the polls, and its precisely why Hillary lost in 2016. If you want your proffered candidate to win, you actually have to convince people that they are worth voting for. And unlike Kamala, Trump is out there doing that. Stein is out there doing that. Chase Oliver is actually doing that (you don’t know who that is do you?), and guess what? Oliver is beating Stein in most swing states.
The claim that Stein is spoiling when they are polling at literally less than measurable numbers is so obviously idiotic, no one worth respecting would give it anything more than a cursory swipe.
There is a meme going around blaming Jill Stein for “spoiling” the 2016 race. I was developing an relatively simple analysis to show how and why its ridiculous to propose that Jill Stein “spoiled” the 2016 election. Specifically, in no race did the green party candidate get more votes than the libertarian candidate. A great example is the headline meme that was up about a day ago here: https://lemmy.world/post/21038666?scrollToComments=true
https://www.politico.com/2016-election/results/map/president/
So in Michigan, ~50k voters went to Stein. In that race, 170k went to Gary Johnson.
Pennsylvania, 48,912 to Stein, and for Gary Johnson, 142,653.
Wisconsin, 30,980 to Stein, and for Gary Johnson, 106,442.
So taking these three as an example, in none of these races, if you were to ‘remove’ the 3rd party candidates, would Hillary have won.
Likewise, the meme assumes that “all” of Green Party voters go to Hillary, and some how the Gary Johnson voters just evaporate.
It doesn’t really make sense at any level. Its part of a broader pattern of voter intimidation that seems to be mostly focused on defending a candidate that has been shown to be lacking, not at all different than what we saw in 2016. I didn’t bother finishing the analysis after a very brief look at the data, because it was so patently absurd to suggest that Jill Stein spoiled anything for anyone in 2016, when she literally did not beat Johnson in a single race.
Imagine buying a policitian’s cryptocurrency
Click on all busses:
There is a short story in here about someone who can’t pass a captcha, loses their identity, and has to move on to becoming a fisherman in Norway.
Dude I can’t even pass a catchpa these day. I still don’t know if a e-bike is a scooter, a bike, or a moped.
#they knew