
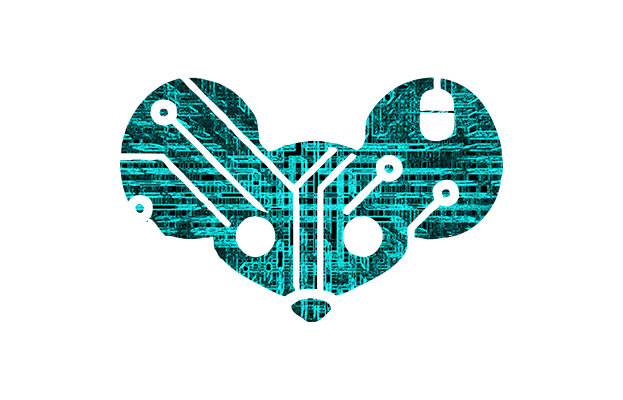
Hah. Snake oil vendors will still sell snake oil, CEO will still be dazzled by fancy dinners and fast talking salesmen, and IT will still be tasked with keeping the crap running.
Hah. Snake oil vendors will still sell snake oil, CEO will still be dazzled by fancy dinners and fast talking salesmen, and IT will still be tasked with keeping the crap running.
This has a lot of “I can use the bus perfectly fine for my needs, so we should outlaw cars” energy to it.
There are several systems, like firewalls , switches, routers, proprietary systems and so on that only has a manual process for updating, that can’t be easily automated.
That’s because they don’t see the letters, but tokens instead. A token can be one letter, but is usually bigger. So what the llm sees might be something like
When seeing it like that it’s more obvious why the llm’s are struggling with it
In many cases the key exchange (kex) for symmetric ciphers are done using slower asymmetric ciphers. Many of which are vulnerable to quantum algos to various degrees.
So even when attacking AES you’d ideally do it indirectly by targeting the kex.
I generally agree with your comment, but not on this part:
parroting the responses to questions that already existed in their input.
They’re quite capable of following instructions over data where neither the instruction nor the data was anywhere in the training data.
They’re completely incapable of critical thought or even basic reasoning.
Critical thought, generally no. Basic reasoning, that they’re somewhat capable of. And chain of thought amplifies what little is there.
No, all sizes of llama 3.1 should be able to handle the same size context. The difference would be in the “smarts” of the model. Bigger models are better at reading between the lines and higher level understanding and reasoning.
Wow, that’s an old model. Great that it works for you, but have you tried some more modern ones? They’re generally considered a lot more capable at the same size
Increase context length, probably enable flash attention in ollama too. Llama3.1 support up to 128k context length, for example. That’s in tokens and a token is on average a bit under 4 letters.
Note that higher context length requires more ram and it’s slower, so you ideally want to find a sweet spot for your use and hardware. Flash attention makes this more efficient
Oh, and the model needs to have been trained at larger contexts, otherwise it tends to handle it poorly. So you should check what max length the model you want to use was trained to handle
What do you think is “weight”?
You can call that confidence if you want, but it got very little to do with how “sure” the model is.
It just has to stop the process if the statistics don’t not provide enough to continue with confidence. If the data is all over the place and you have several “The capital of France is Berlin/Madrid/Milan”, it’s measurable compared to all data saying it is Paris. Not need for any kind of “understanding” of the meaning of the individual words, just measuring confidence on what next word should be.
Actually, it would be "The confidence of token Th is 0.95, the confidence of S is 0.32, the confidence of … " and so on for each possible token, many llm’s have around 16k-32k token vocabulary. Most will be at or near 0. So you pick Th, and then token “e” will probably be very high next, then a space token, then… Anyway, the confidence of the word “Paris” won’t be until far into the generation.
Now there is some overseeing logic in a way, if you ask what the capitol of a non existent country is it’ll say there’s no such country, but is that because it understands it doesn’t know, or the training data has enough examples of such that it has the statistical data for writing out such an answer?
IDK what did you do, but slm don’t really hallucinate that much, if at all.
I assume by SLM you mean smaller LLM’s like for example mistral 7b and llama3.1 8b? Well those were the kind of models I did try for local RAG.
Well, it was before llama3, but I remember trying mistral, mixtral, llama2 70b, command-r, phi, vicuna, yi, and a few others. They all made mistakes.
I especially remember one case where a product manual had this text : “If the same or a newer version of <product> is already installed on the computer, then the <product> installation will be aborted, and the currently installed version will be maintained” and the question was “What happens if an older version of <product> is already installed?” and every local model answered that then that version will be kept and the installation will be aborted.
When trying with OpenAI’s latest model at that time, I think 4, it got it right. In general, about 1 in ~5-7 answers to RAG backed questions were wrong, depending on the model and type of question. I could usually reword the question to get the correct answer, but to do that you kinda already have to know the answer is wrong. Which defeats the whole point of it.
Temperature 0 is never used
It is in some cases, where you want a deterministic / “best” response. Seen it used in benchmarks, or when doing some “Is this comment X?” where X is positive, negative, spam, and so on. You don’t want the model to get creative there, but rather answer consistently and always the most likely path.
https://learnprompting.org/docs/intermediate/chain_of_thought
It’s suspected to be one of the reasons why Claude and OpenAI’s new o1 model is so good at reasoning compared to other llm’s.
It can sometimes notice hallucinations and adjust itself, but there’s also been examples where the CoT reasoning itself introduce hallucinations and makes it throw away correct answers. So it’s not perfect. Overall a big improvement though.
Microsoft’s Dolphin and phi models have used this successfully, and there’s some evidence that all newer models use big LLM’s to produce synthetic data (Like when asked, answering it’s ChatGPT or Claude, hinting that at least some of the dataset comes from those models).
randomly sampled.
Semi-randomly. There’s a lot of sampling strategies. For example temperature, top-K, top-p, min-p, mirostat, repetition penalty, greedy…
As with any statistics you have a confidence on how true something is based on your data. It’s just a matter of putting the threshold higher or lower.
You just have to make so if that level of confidence is not reached it just default to a “I don’t know answer”. But, once again, this will make the chatbots seem very dumb as they will answer with lots of “I don’t know”.
I think you misunderstand how LLM’s work, it doesn’t have a confidence, it’s not like it looks at it’s data and say “hmm, yes, most say Paris is the capital of France, so that’s the answer”. It “just” puts weight on the next token depending on it’s internal statistics, and then one of those tokens are picked, and the process start anew.
Teaching the model to say “I don’t know” helps a bit, and was lauded as “The Solution” a year or two ago but turns out it didn’t really help that much. Then you got Grounded approach, RAG, CoT, and so on, all with the goal to make the LLM more reliable. None of them solves the problem, because as the PhD said it’s inherent in how LLM’s work.
And no, local llm’s aren’t better, they’re actually much worse, and the big companies are throwing billions on trying to solve this. And no, it’s not because “that makes the llm look dumb” that they haven’t solved it.
Early on I was looking into making a business of providing local AI to businesses, especially RAG. But no model I tried - even with the documents being part of the context - came close to reliable enough. They all hallucinated too much. I still check this out now and then just out of own interest, and while it’s become a lot better it’s still a big issue. Which is why you see it on the news again and again.
This is the single biggest hurdle for the big companies to turn their AI’s from a curiosity and something assisting a human into a full fledged autonomous / knowledge system they can sell to customers, you bet your dangleberries they try everything they can to solve this.
And if you think you have the solution that every researcher and developer and machine learning engineer have missed, then please go prove it and collect some fat checks.
The fix is not that hard, it’s a matter of reputation on having the chatbot answer “I don’t know” when the confidence on an answer isn’t high enough.
This has been tried, it’s helping but it’s not enough by itself. It’s one of the mitigation steps I was thinking of. And companies do work very hard to reduce hallucinations, just look at Microsoft’s newest thing.
From that article:
“Trying to eliminate hallucinations from generative AI is like trying to eliminate hydrogen from water,” said Os Keyes, a PhD candidate at the University of Washington who studies the ethical impact of emerging tech. “It’s an essential component of how the technology works.”
Text-generating models hallucinate because they don’t actually “know” anything. They’re statistical systems that identify patterns in a series of words and predict which words come next based on the countless examples they are trained on.
It follows that a model’s responses aren’t answers, but merely predictions of how a question would be answered were it present in the training set. As a consequence, models tend to play fast and loose with the truth. One study found that OpenAI’s ChatGPT gets medical questions wrong half the time.
It’s an inherent negative property of the way they work. It’s a problem, but not a bug any more than the result of a car hitting a tree at high speed is a bug.
Calling it a bug indicates that it’s something unexpected that can be fixed, and as far as we know it can’t be fixed, and is expected behavior. Same as the car analogy.
The only thing we can do is raise awareness and mitigate.
Well, It’s not lying because the AI doesn’t know right or wrong. It doesn’t know that it’s wrong. It doesn’t have the concept of right or wrong or true or false.
For the llm’s the hallucinations are just a result of combining statistics and producing the next word, as you say. From the llm’s “pov” it’s as real as everything else it knows.
So what else can it be called? The closest concept we have is when the mind hallucinates.
This is a very simple one, but someone lower down apparently had issue with a script like this:
https://i.imgur.com/wD9XXYt.png
I tested the code, it works. If I was gonna change anything, probably move matplotlib import to after else so it’s only imported when needed to display the image.
I have a lot more complex generations in my history, but all of them have personal or business details, and have much more back and forth. But try it yourself, claude have a free tier. Just try to be clear in the prompt what you want. It might surprise you.
What llm did you use, and how long ago was it? Claude sonnet usually writes pretty good python for smaller scripts (a few hundred lines)
https://y.yarn.co/2a4fe37e-ed9d-448b-af12-48a7a3202fa5_text.gif